Refined AI approach improves noninvasive BCI performance
Achieving a noteworthy milestone to advance noninvasive brain-controlled interfaces, researchers used AI technology to improve the decoding of human intention and control a continuously moving virtual object all by thinking about it, with unmatched performance.
Pursuing a viable alternative to invasive brain-computer interfaces (BCIs) has been a continued research focus of Carnegie Mellon University’s He Lab. In 2019, the group used a noninvasive BCI to successfully demonstrate, for the first time, that a mind-controlled robotic arm had the ability to continuously track and follow a computer cursor. As technology has improved, their AI-powered deep learning approach has become more robust and effective. In new work published in PNAS Nexus, the group demonstrates that humans can control continuous tracking of a moving object all by thinking about it, with unmatched performance.
Noninvasive BCIs bring a host of advantages, in contrast to their invasive counterparts (think Neuralink or Synchron). These include increased safety, cost-effectiveness, and an ability to be used by numerous patients, as well as the general population. Amid these benefits, however, noninvasive BCIs face challenges because their recordings are less accurate and difficult to interpret.
In He’s recent study, a group of 28 human participants were given a complex BCI task to track an object in a two-dimensional space all by thinking about it. During the task, an electroencephalography (EEG) method recorded their activity, from outside the brain. Using AI to train a deep neural network, the He group then directly decoded and interpreted human intentions for continuous object movement using the BCI sensor data. Overall, the work demonstrates the excellent performance of non-invasive BCI for a brain-controlled computerized device.
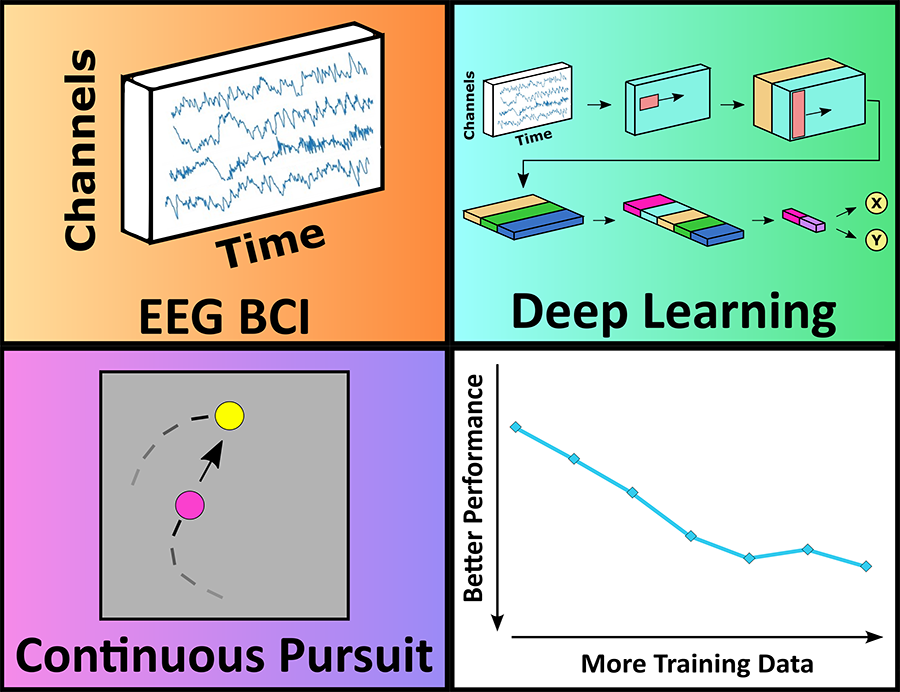
Source: He Lab
“The innovation in AI technology has enabled us to greatly improve the performance versus conventional techniques, and shed light for wide human application in the future,” expressed Bin He, professor of biomedical engineering at Carnegie Mellon University.
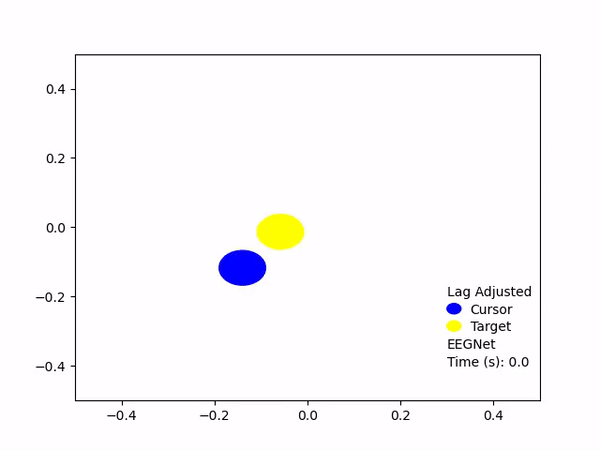
Source: He Lab
This video shows the cursor and target trajectories throughout a single 60-second trial with lag adjusted. The randomly moving target is represented by a yellow circle, while the cursor that the subject controlled using the BCI system is shown as a blue circle. A human subject controlled the blue cursor using the deep learning-based BCI decoder just by thinking about it.
Moreover, the capability of the group’s AI-powered BCI suggests a direct application to continuously controlling a robotic device.
“We are currently testing this AI-powered noninvasive BCI technology to control sophisticated tasks of a robotic arm,” said He. “Also, we are further testing its applicability to not only able-body subjects, but also stroke patients suffering motor impairments.” In a few years, this may lead to AI-powered assistive robots becoming available to a broad range of potential users.
To this end, motor-impaired patients who are suffering from spinal cord injury, stroke, or other movement impairment, but do not want to receive an implant, stand to benefit immensely from research in this vein. “We keep pushing noninvasive neuroengineering solutions that can help everybody,” added He.
This work was supported in part by the National Institute of Neurological Disorders and Stroke, National Center for Complementary and Integrative Health, and National Institute of Biomedical Imaging and Bioengineering.
Other collaborators on the PNAS Nexus paper include the first authors Dylan Forenzo, BME Ph.D. student, and Hao Zhu, former BME Masters student; Jenn Shanahan, former lab technician; and Jaehyun Lim, BME undergraduate student.