Data-frugal deep learning optimizes microstructure imaging
Compared to other computer-vision methods, Elizabeth Holm’s approach to characterizing material microstructure requires only 30-50 images to save researchers an abundance of time and money.
Most often, we recognize deep learning as the magic behind self-driving cars and facial recognition, but what about its ability to safeguard the quality of the materials that make up these advanced devices? Elizabeth Holm and Bo Lei have adopted computer vision methods for microstructural images that not only require a fraction of the data deep learning typically relies on but can save materials researchers an abundance of time and money.
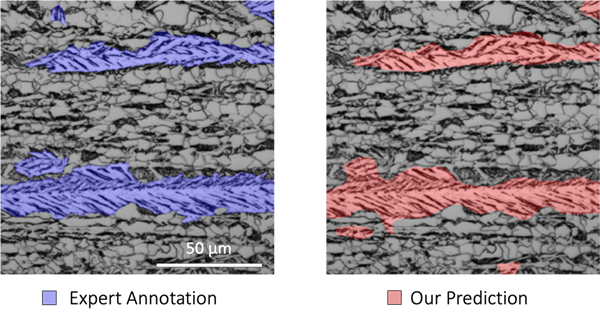
Source: College of Engineering
Annotation performed by an expert vs. deep learning predicted annotation.
Quality control in materials processing requires the analysis and classification of complex material microstructures. For instance, the properties of some high-strength steels depend on the amount of lath-type bainite in the material. However, the process of identifying bainite in microstructural images is time-consuming and expensive as researchers must first use two types of microscopy to take a closer look and then rely on their own expertise to identify bainitic regions. “It’s not like identifying a person crossing the street when you’re driving a car,” explained Holm, a professor of materials science and engineering. “It’s very difficult for humans to categorize, so we will benefit a lot from integrating a deep learning approach.”
Their approach is very similar to that of the wider computer-vision community that drives facial recognition. The model is trained on existing material microstructure images to evaluate new images and interpret their classification. While companies like Facebook and Google train their models on millions or billions of images, materials scientists rarely have access to even 10,000 images. Therefore, it was vital that Holm and Lei use a “data-frugal method,” and train their model using only 30-50 microscopy images. “It’s like learning how to read,” Holm explained. “Once you’ve learned the alphabet you can apply that knowledge to any book. We are able to be data-frugal in part because these systems have already been trained on a large database of natural images.”
In collaboration with German institutes, Holm and Lei, a doctoral student in materials science and engineering, tried different deep learning approaches of laith-bainite segmentation in complex-phase steel. They achieved accuracies of 90%, rivaling segmentation performed by experts. As part of this collaboration, Holm received a grant from the German Research Foundation (DGM) that supports her German collaborators visiting Pittsburgh in early 2022 to work alongside her team.
Additionally, the team is focused on developing an even more frugal deep learning method that would require only one image to get the same results. Aside from steel, Lei has been working with a variety of experimental groups that study deep learning characterization on a variety of materials.
With such promising results, we’ll hopefully be able to introduce this method to a broader community in materials science and microstructure characterization.
Elizabeth Holm, Professor, Materials Science and Engineering
Holm believes, “With such promising results, we’ll hopefully be able to introduce this method to a broader community in materials science and microstructure characterization.”
This research was published in Nature Communications and conducted in collaboration with Fraunhofer Institute for Mechanics of Materials, Karlsruhe Institute of Technology, University of Freiburg, Saarland University, and Material Engineering Center Saarland.