3D printing soft materials with AI
Carnegie Mellon University researchers created a machine learning algorithm that optimizes parameters of soft materials 3D printing. They were able to print 2.5 times as fast and print with an ink that previously didn’t work well.
Additive manufacturing, also known as 3D printing, is a burgeoning technology increasingly being leveraged in the biomedical space. And it’s not just for devices—3D printing is also increasingly being used to print organic tissues and soft materials, such as elastomers.
While 3D printing soft materials, such as with silicone or proteins, offers many distinct advantages, it also introduces many new and complicated variables to consider when creating a new part or material. A team of researchers at Carnegie Mellon University has developed a hierarchical machine learning (HML) process that takes these variables into account and optimizes the outcomes when 3D printing silicone, using Freeform Reversible Embedding (FRE).
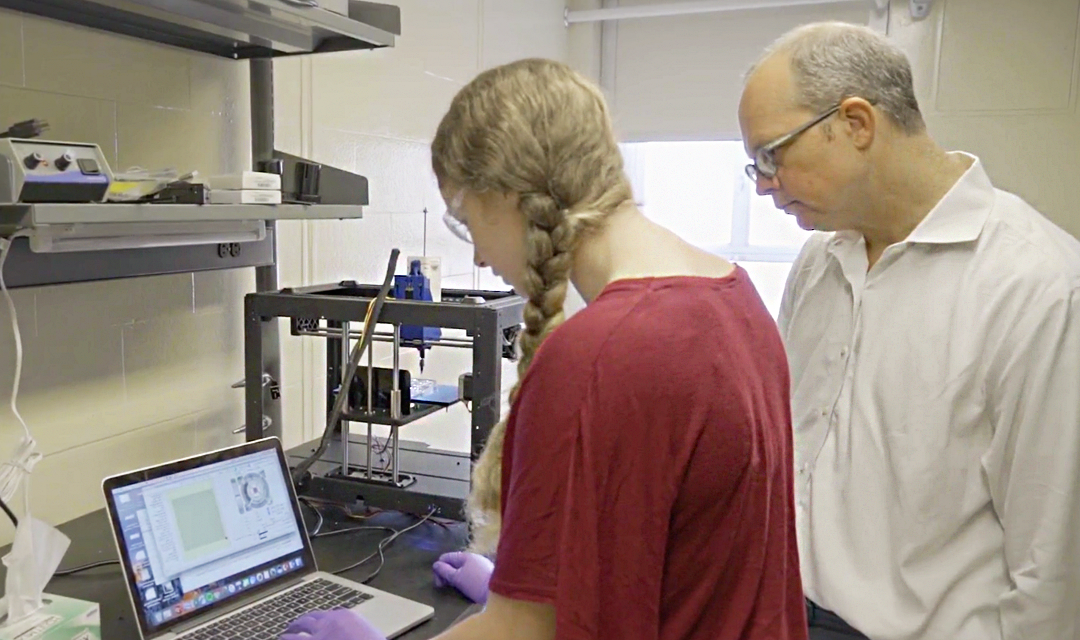
Source: College of Engineering
Professor Newell Washburn and a student print a soft materials component in the lab.
The FRE 3D printing process depends on several different variables including the materials, how the materials are formulated, and the printing process itself. In these circumstances, users might choose these variables based on intuition, which would just be a guess based on past experience, or iteratively: changing one variable at a time until they find the optimal setting for each parameter. In this way, the user thinks about how these components interact with each other. But that doesn’t always lead to the fastest or most optimal result.
“Increasingly, we’re starting to make more soft material-based components and devices for low-tech areas such as sensors and medical applications, and for high tech areas like soft robotics,” said Newell Washburn, professor of biomedical engineering and chemistry at Carnegie Mellon. “But every time you add another component you exponentially raise the complexity of the manufacturing process. That’s where a machine learning algorithm could really help with optimization.”
Every time you add another component you exponentially raise the complexity of the manufacturing process. That’s where a machine learning algorithm could really help with optimization.
Newell Washburn, Associate Professor, Biomedical Engineering and Chemistry
Washburn and his colleagues Adam Feinberg in biomedical engineering (whose lab developed FRE 3D printing), Barnabas Poczos in the Machine Learning Department, and materials science and engineering Ph.D. student Aditya Menon recently published a paper on using HML to optimize soft materials 3D printing. The study was published in the journal 3D Printing and Additive Manufacturing.
Machine learning algorithms, which are a type of artificial intelligence algorithm, are designed to develop a relationship between the input variables and the outputs of a complex system based on training data. Traditional machine learning algorithms operate like black boxes where the algorithm will try to generate a rule to connect the input and output based on training data, but it may not be because of any physical correspondence. For a complex process, you need a lot of parameters in the model to make the connection between input and output, meaning you need tons of data.
But for the complex problem that the research team is working on, there isn’t a lot of data. To account for this, the team custom-built an HML algorithm that incorporates expert knowledge about how the physical systems and parameters operate, rather than working like a black box. Traditional machine learning systems have layers of nodes that connect the input and the output without understanding this information. Instead, HML uses knowledge of the underlying physical system—in this case the physics of the FRE 3D printing variables—to draw connections between the right variables, significantly cutting time and the amount of data needed.
The algorithm selects optimal parameters for variables in different categories: materials, or what they choose to print with; formulation, or how the materials are mixed; and process, including the flow rate, the speed at which the needle moves with other instrument, or manufacturing parameters. Every variable interacts with the others, creating a complex design space.
“Essentially what we’re doing is we’re building a digital twin, or a digital replica, of both the physical process and our human conceptual understanding of the process to achieve optimal results,” said Washburn.
While optimization done with the algorithm did not increase print fidelity, it increased print speed by two-and-a-half times and enabled the researchers to print using an ink that previously hadn’t worked well. The algorithm also identified a unique silicone formulation and printing parameters that had not been found previously through trial-and-error approaches.
With these results, the researchers—some of whom are affiliated with both the Manufacturing Futures Initiative and the Bioengineered Organs Initiative—are optimistic that HML will have broad application for planning and optimizing in the 3D printing of many types of soft materials, for biomedical applications among others.