Machine learning and extended reality used to train welders
Researchers apply machine learning to a lightly-modified off-the-shelf welding helmet and torch integrated with a Meta Quest headset and controller to train welders.
Ever since the ancient Egyptians hammered two pieces of gold together until they fused, the art of welding has continuously progressed. Iron Age blacksmiths used heat to forge and weld iron. The discovery of acetylene in the early days of the industrial revolution added a versatile new fuel to welding. At the end of the 19th century, two engineers invented metal arc welding, and more recently, the rise of robotic welding systems and advances in high-strength alloys expanded welding applications.
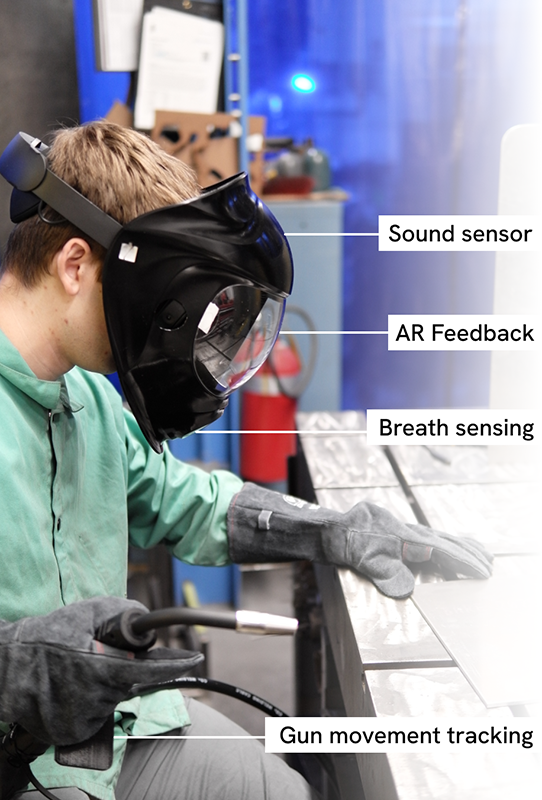
Source: College of Engineering
A welder uses the gun to place coordinate locations for the start and end of the weld, linking the real world weld line to a graphic representation in the XR display.
Despite the long history of welding technology improvements, it remains a challenging skill to learn. Welding requires a combination of technical knowledge and manual dexterity. And given its prevalence throughout numerous industries, including manufacturing, construction, aerospace, and automotive, the need for skilled welders remains strong. According to the American Welding Society, the growing shortage of welders means U.S. employers are now facing a deficit of 375,000 welders.
At Carnegie Mellon University, researchers are addressing the problem by developing a new way to train welders that once again applies an emerging technology. With financial support from the Manufacturing Futures Institute (MFI) seed funding program, Dina El-Zanfaly, an assistant professor in the School of Design, and Daragh Byrne, an associate teaching professor in the School of Architecture worked with a team of researchers to develop an extended reality (XR) welding helmet and torch system to help welders acquire the embodied knowledge they need to master the challenging skill.
“Not only is this a really cool project, but it also incorporates the key objectives of the MFI mission,” said Sandra DeVincent Wolf, the executive director of MFI. “It is groundbreaking research that advances manufacturing technology, contributes to workforce development, and engages partners from the local community.”
Extended reality combines virtual reality (VR), which is a computer-generated environment that simulates a realistic or imaginary experience; augmented reality (AR), which combines computer-generated information with a user’s real-world environment; and mixed reality (MR), where real-world and digital objects coexist and interact in real-time. Together, these features create an immersive experience that allows users to interact with information, environments, and digital content in real-time.
Training welders requires the development of hand-eye coordination and a keen perception of the position and movement of the body in space. This embodied knowledge is acquired through hands-on interactions with tools, and materials and can be difficult to replicate in training scenarios.
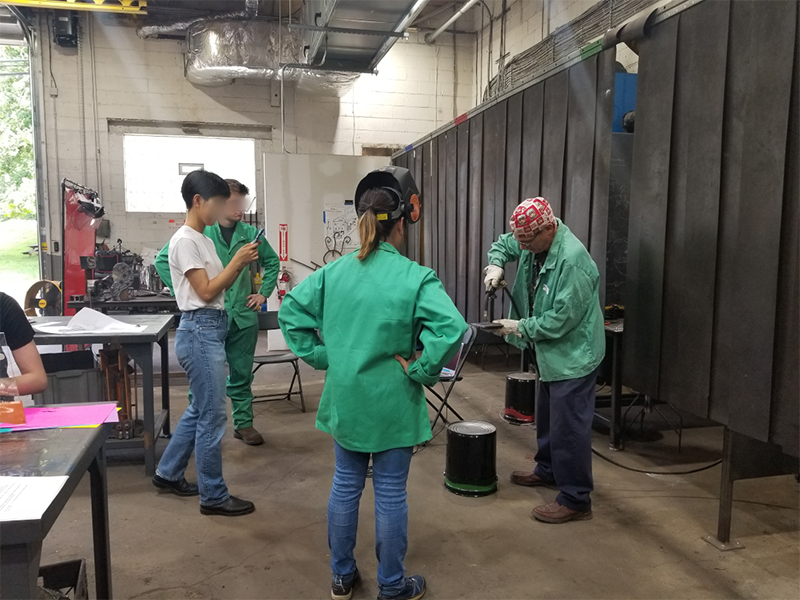
Source: College of Engineering
An instructor from the Industrial Arts Workshop demonstrates proper welding technique to Carnegie Mellon students.
The Carnegie Mellon researchers worked to better understand the training challenges by organizing a series of co-design workshops. They worked with eight instructors and four students at the Industrial Arts Workshop (IAW), a non-profit youth welding training program in the Hazelwood neighborhood of Pittsburgh, to develop a system that integrates a welding helmet and torch with a Meta Quest Pro and a machine learning model that enhances the embodied learning of welding in three key ways.
Visual XR guides and integrated motion sensing
The highly-immersive and embodied nature of welding practice makes it exceptionally difficult for an instructor to visually monitor the process and provide feedback to the student in a timely, safe, and audible manner while they are welding. Also, neither written instruction nor feedback can convey the nuanced hands-on skill in real-time.
The researchers overcame these obstacles by modifying a welding helmet with a Meta Quest headset that displays a series of visual feedback mechanisms that guide the welding student during training sessions and provides a record of their performance that instructors can assess during the session or after it is completed.
Two separate XR indicators within the welding helmet show the slight changes and adjustments the welding student should make to maintain the correct angle of the welding gun that is connected to the Quest Touch controller. The status icons, which are near the top of the headset viewport, allow users to see the feedback without taking their focus away from the active weld. The status icons also give a much clearer overview of performance for instructors and users while viewing the live playback.
The researchers leveraged feedback from the workshop instructors to determine how to calibrate the XR representation of the weld to a real workpiece so that users can set the start and endpoints of the weld line with the welding gun to set the position of the scrolling guideline they need to follow.
Not only is this a really cool project, it also incorporates the key objectives of the MFI mission.
Sandra DeVincent Wolf, Executive Director, Manufacturing Futures Institute
Sensing sonic cues during welding practice
The Carnegie Mellon researchers learned from workshop instructors that experienced welders are able to assess welds through active listening. So instead of evaluating welds visually after they are completed, their system can use an auditory-based method to diagnosis the weld in real time.
“For example, a good welding speed should sound like sizzling bacon, not popcorn according to the instructors,” explained El-Sanfaly.
Metal Inert Gas (MIG) welding involves extruding a metal wire through the tip of the welding gun, shielding the wire with inert gas, and using the heat generated by short-circuit current between the wire and the workpiece to fuse the two metals together. Incorrect settings of this system result in poor-quality welds. For example, if the amperage of the welder is set too low, it will result in an excessively thin weld bead and lead to inconsistent penetration of the working plate.
According to prior research and feedback from the AIW instructors, different settings result in changes of the welding sound, which offers potentially important training feedback. But the extreme heat, light, and sound conditions in the welding space together with the bulky welding helmet and other personal protective gear needed to guard against the intense heat, sparks, ultraviolet radiation, and metal splatter, limits the welder’s ability to perceive this auditory stimulus.
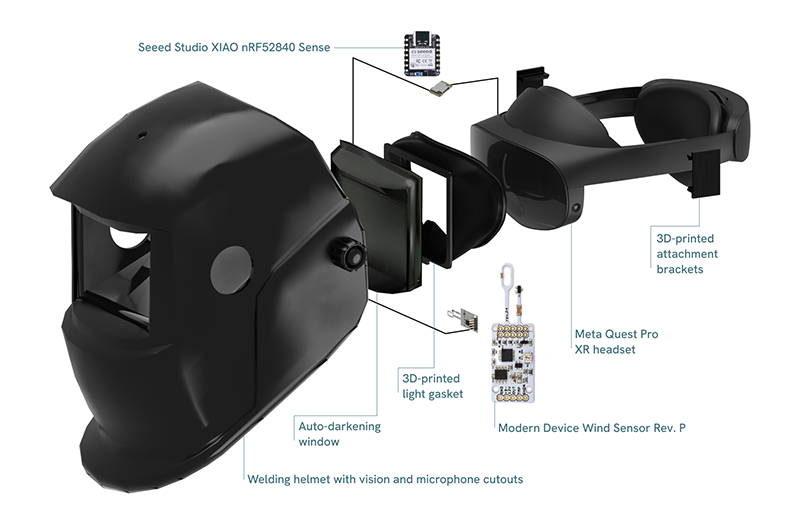
Source: College of Engineering
The researchers modified the welding helmet by fixing it to the Meta Quest Pro XR headset and interfaced the Seeed ESP32S3 board to a Unity software program running the XR display, using the Quest Pro’s USB-C port and the Serial Port Utility Pro plugin. The adjustable Quest head strap and connected battery replace the traditional helmet insert, and additional components were 3D printed with PLA filament.
By employing Tiny Machine Learning (TinyML) enabled sound detection to recognize key factors such as settings and tip distance, the researchers trained and deployed their machine learning model to provide visual feedback to indicate errors that are detected by sounds such as those that are made when the tip of the gun is being held too far away from the welding plate.
The researchers asked experienced welders to repeatedly perform the same welding movement, only changing one setting per weld. They collected more than 20 minutes of audio data, evenly distributed across the category settings to use in training and testing a TinyML classification model.
TinyML focuses on deploying and running machine learning models on resource-constrained devices, such as a microcontroller, which in this case was connected to the augmented helmet to provide feedback. The researchers trained the TinyML model to alert trainees to attend to common errors, such as incorrect settings and gun tip distance.
Sound was also used to detect the beginning and end of welds. Researchers collected nineteen minutes of welding sound using recordings from five different devices simultaneously: two microcontrollers, two Samsung smartphones, and a USB microphone, to train a classification system that could detect welding with 97% accuracy. This classifier replaced the need to electromechanically detect interactions with the physical button on the welding gun, which was used to initiate welding tracking and to make the system more portable.
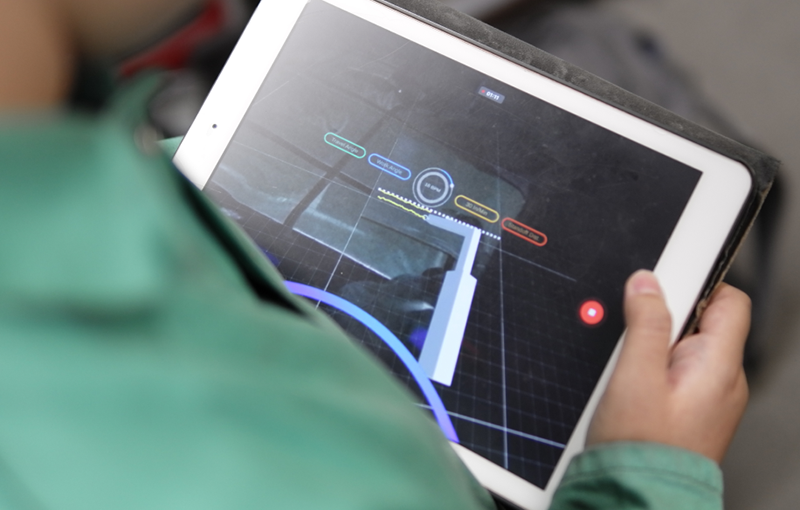
Source: College of Engineering
Realtime instructor view allows each student’s live performance to be cast to the instructor’s tablet. An instructor or student can review real-time or recorded point-of-view footage of the experience to monitor behaviors or analyze difficult scenarios.
Pre-welding meditation
During the workshops, researchers saw that instructors encouraged students to use mediation and breathing exercises before starting to weld as a way to induce relaxation and foster a sense of focus to offset the effects of the welding environment, which can be overwhelming due to loud noises, sparks, heat, and burning smells.
In order to enhance breathing exercises and mindfulness practices, the researchers programmed the platform to begin each welding session by encouraging trainees to engage in breathing exercises. They also placed an off-the-shelf anemometer near the mouth and nose inside the welding helmet to measure the exhaled wind speed of breath and track the breathing pattern of welders over time in order to develop system prompts to help welding students adopt mindfulness and regulate their breathing that can improve task performance.
The system’s ability to sense motion, detect sound, and enhance users’ focus through mediation and breathing exercises can help students transfer the skills they acquire in the virtual training to actual welding practice. Its ability to provide guidance in real time provides numerous advantages for both students and instructors who otherwise must rely on information derived after a weld is complete to assess the performance. The overall approach could also inform crafts and skill training in XR systems beyond welding training.
A really exciting aspect of our work is the ability of our system to enable in-situ welding experiences using a lightly modified off-the- shelf XR and welding setup.
Dina El-Zanfaly, Assistant Professor, School of Design
“A really exciting aspect of our work is the ability of our system to enable in-situ welding experiences using a lightly modified off-the-shelf XR and welding setup,” explained El-Zanfaly.
Their work has already received recognition—receiving awards at the 2023 Association for Computing Machinery (ACM) Conference on Interactive Surfaces and Spaces and at the 2024 ACM Conference on Tangible, Embedded, and Embodied Interactions.
Moving forward, the team plans to pursue numerous opportunities to improve both the technical dimensions of the work and the embodied experience. They plan to deploy the platform in A/B lab studies and over multiple weeks at IAW to assess how extended use of this device contributes to the formation of skills, habits, and the novice experience.