Escape from the heat island
New models of the urban heat island effect could inform the next generation of urban planners and help prevent hundreds of heat-related deaths occurring every year.
Dangerously high temperatures, especially in cities, often cause conditions such as heat stroke and heat exhaustion or exacerbate preexisting medical conditions such as cardiovascular disease. Between 1999 and 2010, at least 8,081 people died in the United States due to heat-related illnesses.
One of the main factors behind this startling number is the urban heat island effect. This causes high disparities in temperature between cities and surrounding areas due to heat trapped by concentrations of populations and man-made infrastructure. Eighty-one percent of deaths reported—more than 6,500 total—occurred in urban areas.
Armed with a grant from the National Science Foundation’s (NSF) Prediction of and Resilience against Extreme Events (PREEVENTS) program, a team of researchers from Carnegie Mellon set out to provide cities with a tool to better understand this dangerous phenomenon.
The team was lead by Associate Professors Matteo Pozzi and Mario Berges of the Department of Civil and Environmental Engineering, and their Co-PI, research scientist Kelly Klima of the Department of Engineering and Public Policy.
The fruit of their labor is SHADE, which stands for Surface Heat Assessment for Developed Environments. Through SHADE, the team, including postdoctoral researcher Carl Malings and Professor Elie Bou Zeid of Princeton, have developed small-scale spatio-temporal models of Pittsburgh’s urban temperatures.
While researchers at Princeton focused on simulating weather conditions and other factors that contribute to temperatures at a given location, the CMU team took a more data-driven approach. Pozzi and Malings, a Ph.D. student of Pozzi’s at the time, worked to develop “statistical models to better model, understand, and realize how temperature changes across time and space” within the urban environment.
They found that high temperatures caused by regional heat wave events can add yet another potent ingredient to the pot of dangerous temperature factors at play in the urban environment.
“One, the temperature is higher in urban areas,” says Malings. “Two, it's even higher during heat waves. And three, the temperatures can vary a lot throughout the city, because the building composition is very different. This makes the effect very different.”
While the team at Princeton was able to model these factors at a given point, doing so over the entire city would be extremely computationally intensive, making this method impractical for real-time temperature modeling and prediction.
“The idea that we're working on is to build what we call ‘surrogate models,’ that are basically simpler models that capture the same kind of information but can be run much more quickly to get close to real-time forecasts,” says Malings.
Using this system of surrogate models, the team wasable to begin observing larger trends in temperatures between different locations across time. Despite being in the early stages of the project, their findings have already led to several publications, including their most recent paper on a methodology for optimal sensing to support decision-making in scenarios of extreme temperatures. This could allow for a much better early warning system for populations at high risk for heat related illness.
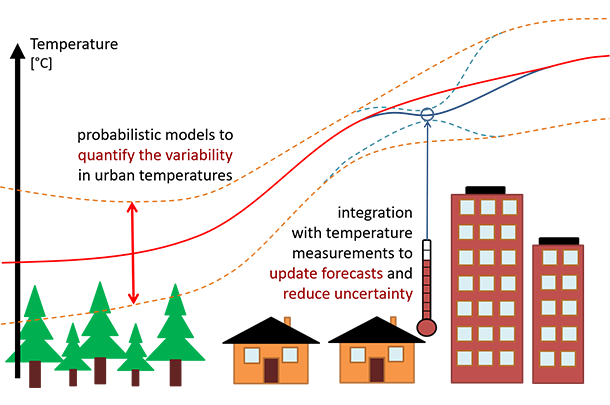
Source: Carl Malings
A diagram of how the different aspects of SHADE are integrated to create more accurate temperature models.
In the long-term perspective, the model could inform infrastructure decisions for civil engineers. Using information provided by the team’s modeling techniques, city planners and architects could integrate green infrastructure and other heat mitigation techniques into the cities of the future, fighting the urban heat island effect at its source.
“The resulting framework will help future urban planners and managers to make decisions involving temperature within the city,” says Berges, “and they’ll be able to take advantage of the rapidly growing stream of sensor data that is available in urban environments."
For Malings, now a postdoctoral researcher in the Department of Mechanical Engineering, the information from SHADE will also help inform future decisions about where to place sensors for optimal results. The inferences he hopes to draw from these sensors are not only limited to the urban heat island effect.
“You can do a similar thing with air pollution, where you take your prior prediction of pollution concentrations and then update those with the sensor measurements and optimize decisions based on that,” says Malings.
Having thus far restricted their modeling to Pittsburgh and New York, the team plans to spread its scope to a larger range of cities in the future.
“Cities have similar properties, but just starting in Pittsburgh and New York as our case studies, there are definitely different behaviors between the two,” says Pozzi. “Our idea for the future is to develop urban scale models for different cities, but some specific calibration needs to be done for each individual city.”
Learn more about Mario Berges’, Matteo Pozzi’s, and Kelly Klima’s research: