Combining experts and automation in 3-D printing
Engineering researchers have developed a novel approach to optimizing soft material 3-D printing. The researchers’ Expert-Guided Optimization method combines expert judgment with an optimization algorithm that efficiently searches combinations of parameters relevant for 3-D printing, enabling high-fidelity soft material products to be printed.
Researchers in Carnegie Mellon University’s College of Engineering have developed a novel approach to optimizing soft material 3-D printing. The researchers’ Expert-Guided Optimization (EGO) method combines expert judgment with an optimization algorithm that efficiently searches combinations of parameters relevant for 3-D printing, enabling high-fidelity soft material products to be printed.
The researchers—which include lead author Sara Abdollahi, a Ph.D. student in biomedical engineering; Adam Feinberg, associate professor of biomedical engineering and materials science and engineering; Alex Davis, assistant professor of engineering and public policy; and Dietrich College of Humanities and Social Sciences Professor John Miller—designed the EGO method to optimize high quality 3-D prints of soft materials.
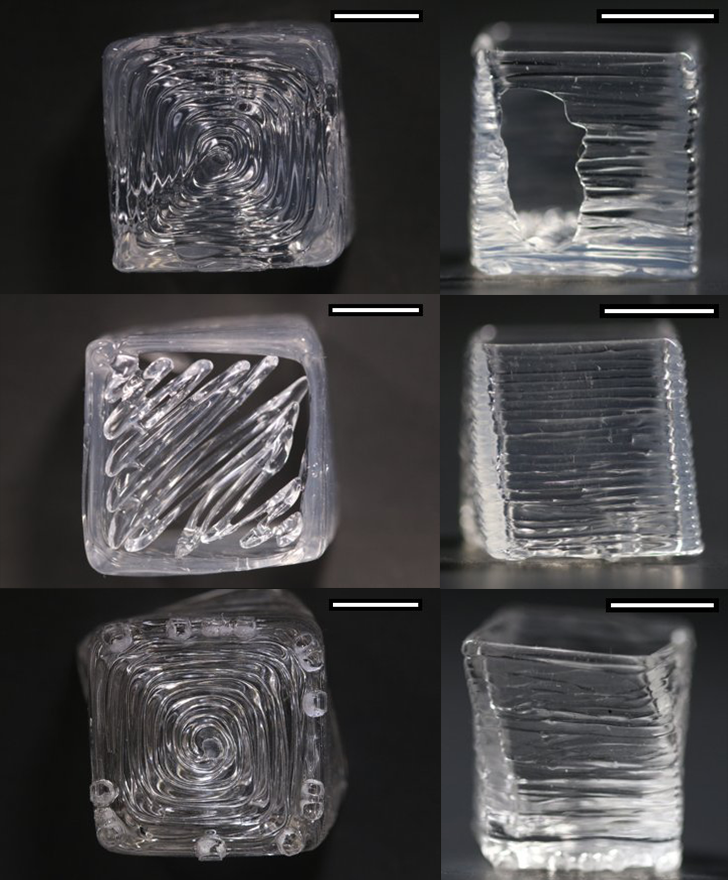
Source: Sara Abdollahi, Alexander Davis, John H. Miller, Adam W. Feinberg
Images of the PDMS 3-D prints made using the S3D CAD slicer to determine toolpath.
In their paper, “Expert-guided optimization for 3D printing of soft and liquid materials,” which was recently published in PLOS One, the researchers demonstrate the EGO method using liquid polydimethylsiloxane (PDMS) elastomer resin, a material often used in wearable sensors and medical devices. The researchers used a printing method called freeform reversible embedding (FRE), in which soft materials are deposited within a gel support bath.
When it comes to 3-D printing soft materials, many parameters can affect the final product. How fast the head of the 3-D printer moves, the consistency of the gel bath in which the product is printed, and the concentrations of each material in the print are just a few of the variables that can affect the final product. In each print, there can be dozens of parameters to take into account, and many more possible combinations of them.
If an experimental material has 20 print parameters with five levels, the experimenter can have trillions of combinations of print settings to explore.
Sara Abdollahi, Ph.D. student, Biomedical Engineering, Carnegie Mellon University
A typical optimization model or experimental design will focus on a few parameters that are considered most important to the print. However, adapting these optimization models for experimental materials, whose 3-D printing characteristics aren’t well known, can be extremely challenging.
“When 3-D printing thermoplastics, if you have just five or 10 main print parameters and want to explore, say, five levels of each, a factorial design can result in millions of possible combinations of settings to print,” says Abdollahi. “The combinations become even more daunting when exploring an experimental material whose print characteristics are unknown. For example, if the experimental material has 20 print parameters with five levels, the experimenter can have trillions of combinations of print settings to explore.”
However, with the EGO model, this challenge can be made less of an obstacle because experts are able to rule out many combinations as being ineffective. By combining an expert’s scientific judgment with efficient search algorithms, EGO significantly reduces the time and energy required to find combinations that yield optimal 3-D prints for experimental materials.
“The purpose of EGO is to create an effective search algorithm that explicitly combines both expert knowledge and traditional search algorithms,” says Davis. “Typically we think of machine learning being useful for big data, but EGO works in situations when we have little or no data and need to rely on expert judgment, then through a combination of search algorithms and the expert’s knowledge, effectively transition from small to big data.”
The EGO model is comprised of three steps. First, a human expert selects the initial set of parameters, giving the algorithm the boundaries for search. Then, a hill climbing algorithm searches within those boundaries for promising combinations of those parameters, resulting in a “local optimum.” Finally, the expert evaluates the local optimum and decides whether to alter the search process by adding new parameters, or continue to search within the existing boundaries. The process iterates until an ideal solution is found.
The EGO method, which can extend beyond the 3-D printing of soft materials for a variety of engineering processes, has great potential as a systematic tool to discover the key parameters that yield reproducible, high-quality, novel materials.