Predicting material behavior using small datasets
Newell Washburn and a team of researchers from CMU have designed a novel machine learning model that predicts and optimizes the properties of complex physical systems using small datasets.
A team of researchers from Carnegie Mellon University including Newell Washburn, associate professor in biomedical engineering and chemistry, have designed a novel model for predicting and optimizing the properties of complex physical systems. Most models of this kind require large datasets, but Washburn and his team have developed a new way to make predictions—a way that requires far smaller amounts of data than usual.
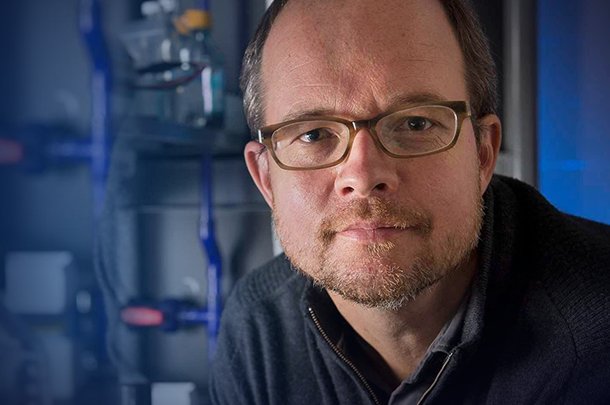
Source: Carnegie Mellon University
Newell Washburn, associate professor in biomedical engineering and chemistry
“Most established methods in machine learning are pretty data-intensive,” says Washburn. “Knowing that, we started thinking about ways you can use what you already have—since you know something about the underlying interactions of the system, are there ways that you could use that prior knowledge to leverage or supplement smaller datasets?”
The method presented in this paper uses machine learning to simplify the process of predicting how complex physical systems will behave. Complex physical systems are networks that exhibit specific behaviors as a result of their many parts’ physical interactions. Suspensions, for example, are considered part of a subset of complex physical systems, called complex fluids—think cement, paint, cosmetics, or even complex biomaterials—in which solid particles known as dispersants are suspended within a liquid.
There’s a growing interest in applying machine learning to science and engineering, but it’s not just the machine that’s learning. The people are learning too.
Newell Washburn, Associate Professor, Biomedical Engineering & Chemistry, Carnegie Mellon University
This research, funded by the National Science Foundation and published in Molecular Systems Design & Engineering, is a novel method of predicting the behaviors of new materials based on a machine learning method that takes input—domain knowledge—from experts in the field. When the researchers give the model known variables and information, the model then learns from that information, applying these “knowns” to new small datasets in order to predict the behaviors of existing and brand-new materials. This model, considered by Washburn to be a tool for developing an understanding of how complex systems work, can be used to optimize existing materials, as well as to predict new materials entirely.
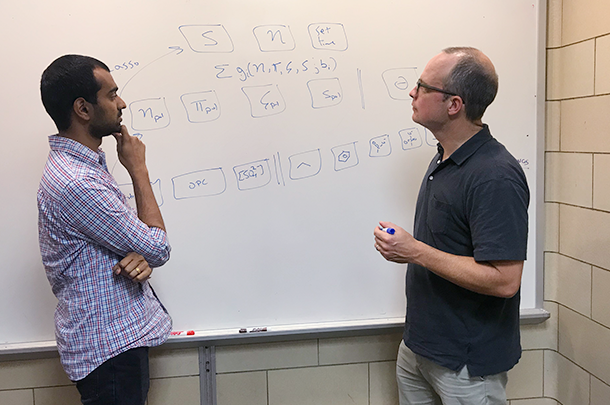
Source: Carnegie Mellon University College of Engineering
Washburn and one of his students discuss the novel predictive model.
“People have been working with things like suspensions and complex fluids for decades, and have developed a really good basic understanding of how they work,” says Washburn. “We wanted to have a model that could leverage a lot of that existing knowledge. The model looks at all of our data—not just the data that agrees with our intuition.”
In their paper, titled “Elucidating multi-physics interactions in suspensions for the design of polymeric dispersants: a hierarchical machine learning approach,” Washburn and his team also describe one of the model’s successful predictions: a brand-new dispersant that could be competitive with the current leading commercial dispersant on the market. When the researchers synthesized this dispersant, it was shown to exhibit similar properties to the leading commercial dispersant, but with a significantly different composition and molecular architecture.
“There’s a growing interest in applying machine learning to science and engineering,” says Washburn, “but we don’t normally invoke the underlying physical laws when we’re building these models. So our goal was to embed this domain knowledge in the model, to help us better understand how these complex physical systems actually work.”
He adds, “It’s not just the machine that’s learning. The people are learning too.”