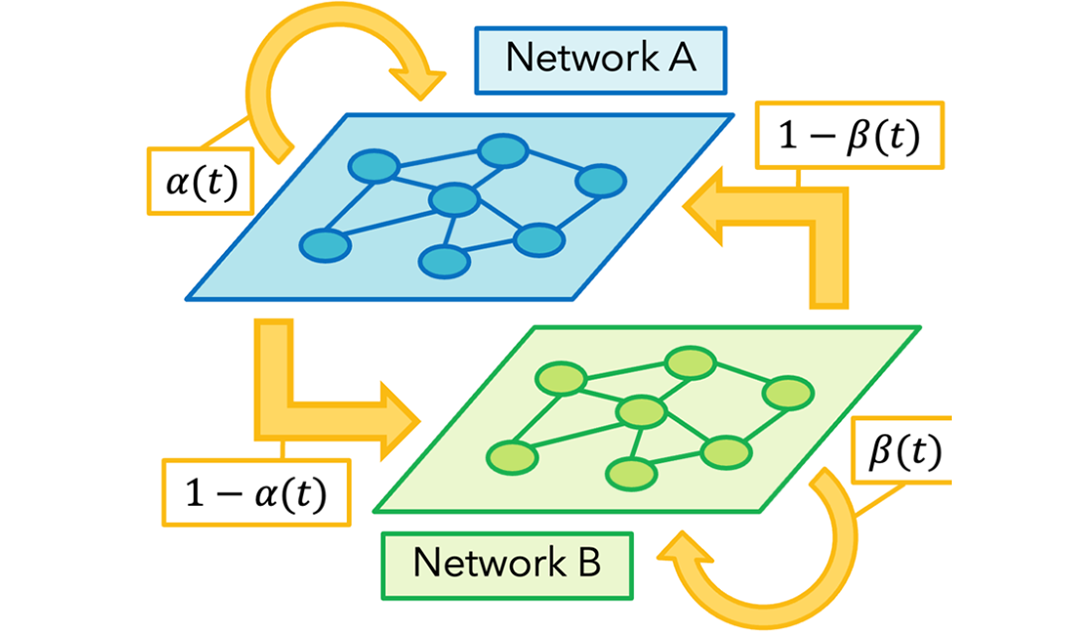
Today’s manufacturing enterprises often rely on increasingly complex supply chains to provide the components they need to make their final products. This complexity and interdependence can render supply chains vulnerable to disruptions. For example, if a machine used to make many parts suddenly slows down and/or requires maintenance, this slowdown might bottleneck other fabrication tasks that use products made by this machine, leading to a cascade of disruptions.
Additive manufacturing may help to mitigate the propagation of such disruptions by offering an alternative fabrication avenue, but the complexity of supply chain relationships makes it difficult to optimize how such alternatives should be utilized in the supply chain.
Our main technical milestones for this project focus on the three core components:
- Designing reinforcement learning methods for scheduling workers to machines in manufacturing settings.
- Quantifying the vulnerability of supply chain networks to points of failure that lead to “cascades” of failures throughout the supply chain network.
- Leveraging our vulnerability analysis to supplement schedules with additive manufacturing components, thus allowing our reinforcement learning algorithms to mitigate vulnerability risks.