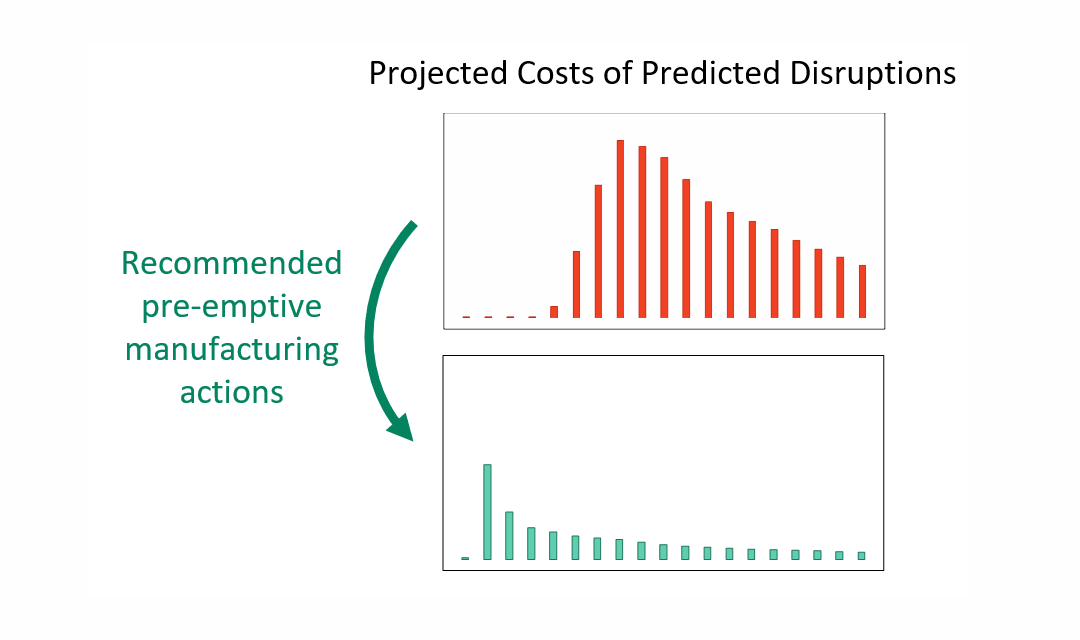
This project creates a digital twin tool that allows manufacturers to monitor possible future supply chain shocks and understand what actions they can take ahead of time to adapt their production lines and product plans to avoid the consequences of the disruption to their operations and sales. This tool provides:
- Synchronized tracking of material flows in the supply chain, from raw minerals to battery cells, with probabilistic future-projected flows determined by the potential of emerging disruptions.
- Machine Learning and Multimedia Analysis (ML-MA) of documents, charts, and visuals in the news and other sources that can be used to forecast potential disruptions in the supply chain. This system dynamically integrates insights and updates, leveraging probabilistic logic programming to anticipate the evolving states of the supply chain.
- Recommended actions for manufacturing that would avert the impacts of the forecasted disruptions, such as rapidly switching production lines to different battery chemistries, or reconfiguring vehicle production lines to accommodate alternate battery packs.
- On-demand exploration of “what-if” analysis of future potential disruption scenarios.