A key challenge to automating metals additive manufacturing (MAM) is identifying the best materials for the need at hand subject to the required properties, defect tolerance, printability, post-processing and cost/availability. While ML is already enabling high-throughput synthesis and characterization in areas of materials discovery where properties can be predicted using first-principles calculations or other readily available surrogates, there is still no centralized repository of organized data for automating synthesis and characterization of complex structural materials such as steels, superalloys, and multi-principal element alloys (MPEAs), though the data is available in an unstructured form, distributed across tables and narrative text describing experimental conditions and findings in the scientific literature. Further, burgeoning work using approaches from the area of natural language processing (NLP), do not easily transfer to new areas, applications, and techniques as they emerge without substantial intervention by human experts to annotate new data for supervised learning, which can be prohibitively costly and time consuming.
Researchers propose to tackle this challenge by developing novel methods at the intersection of ML and NLP capable of automatically populating a structured database of materials and their properties derived from the text and tables of scientific research articles, while minimizing the amount of data annotation required by human experts. Researchers will develop approaches that combine deep learning with active learning and structured prediction to enable:
- high accuracy automated extraction of structured data from the unstructured text of scientific literature with a minimal number of annotated examples
- agile generalization from one area of interest to another. These approaches will be applied to automatically populate a growing database of structured data extracted from scientific literature
The resulting dataset will enable users to query the rich information available in the text of research articles efficiently and effectively and enable the training of ML models capable of automatically proposing solutions to challenges in MAM as they arise.
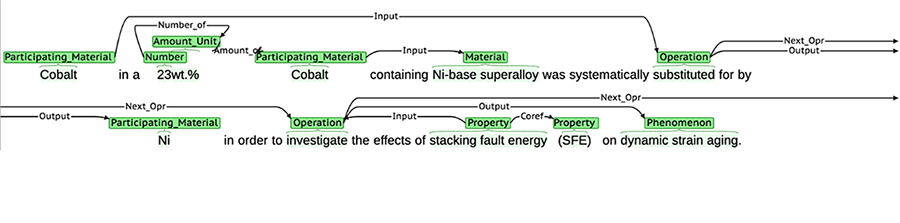