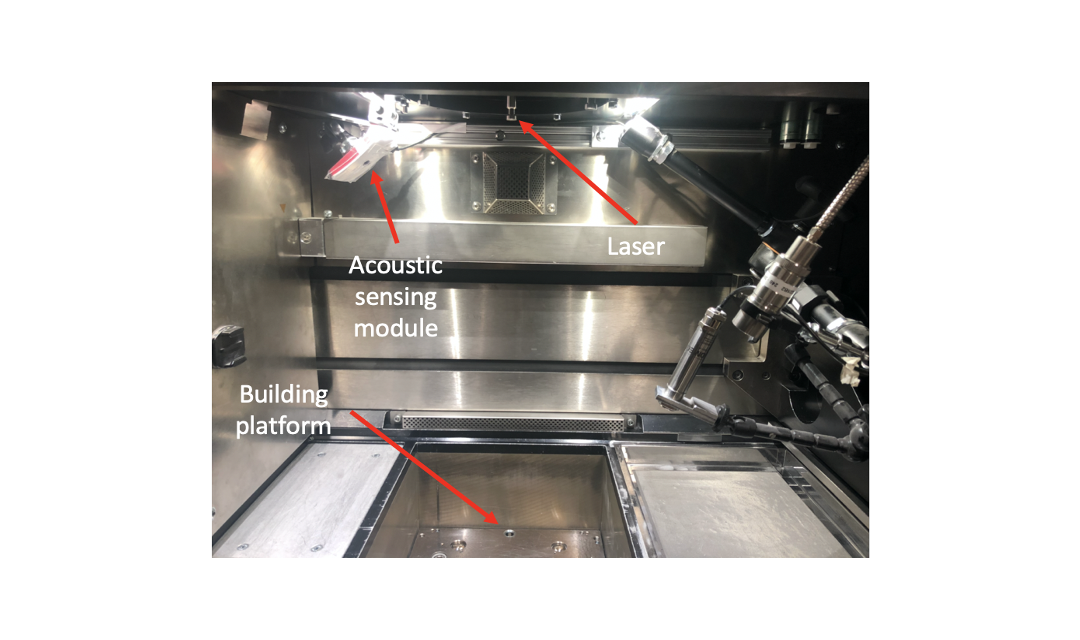
The primary objective in this project is to enable real-time flaw detection in laser powder bed fusion (LPBF) AM technologies through a computerized analysis of acoustic emissions during the build process. Acoustic monitoring of laser/melt pool interactions can enable the identification of conditions yielding flaw formation or builds without flaws. Our work in the first year of this program has indicated clear differences in acoustic signals between various flaw types, including keyholing, bead-up, lack of fusion, and flaw-free builds. Based on labeled acoustic emissions that were collected from our EOS machine, we developed two data-driven classification approaches that can distinguish these four configurations with 97% accuracy. However, hyperparameter optimization revealed that the optimal sliding time window size for classification to be 1.92 seconds, which is significantly longer than typical flaw formation durations. Additionally, the labeled data was obtained by inducing each flaw type continuously over long bead lengths without frequent transitions across the flaw types. In year 2 of this project, we work to build on our experience to investigate how automatic classification with 90% accuracy or better can be achieved for shorter window sizes (5-10ms) where frequent transitions across different flaw types might also be present. The work will involve acquiring and setting up new acoustic sensor hardware, the generation of new training and test data using physical builds, and the development of new machine learning systems. We will use the EOS M290 and the TRUMPF TruPrint 3000 machines as our test beds.