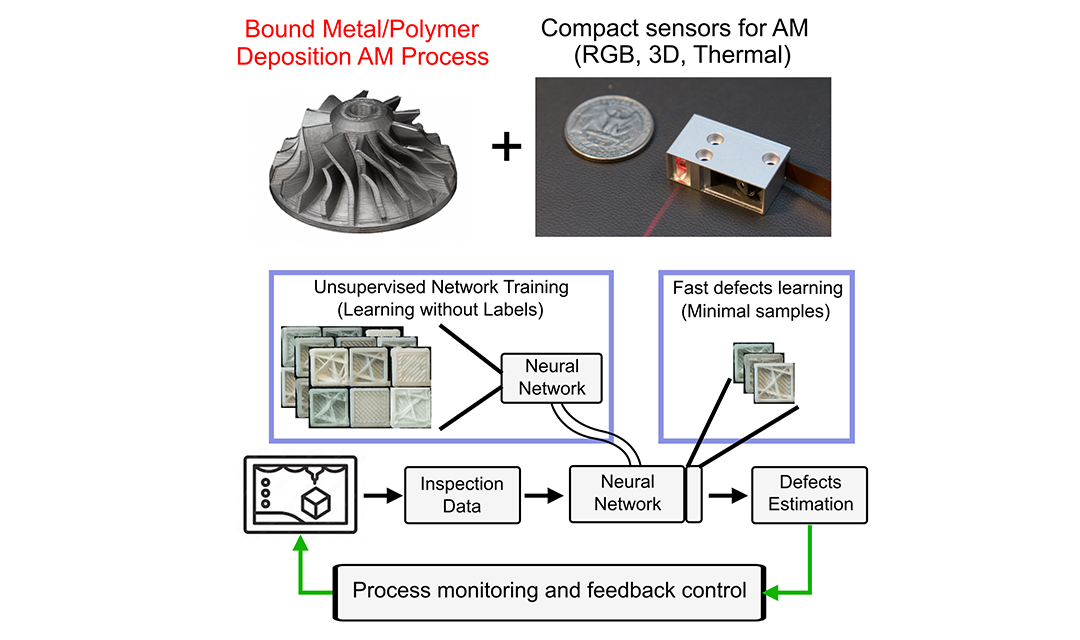
The challenges of applying AI solutions to defect detection in additive manufacturing (AM) tasks lie in two folds: 1) the extreme time costs of data collection and 2) the difficulty of capturing the complete cases of defect types and physical samples. Conventional feed-forward machine learning methods follow a general pattern of learning a relationship between input data (images or measurements during AM processes) and human-provided labels (defects sample datasets). However, this strategy heavily relies on large databases of manually labeled data and fixed pre-defined labels to generate effective learning. Unfortunately, this poses a problem in AM due to the physical process of manufacturing parts for data collection is inherently time-consuming, making the collection of a massive all-encompassing AM defect database infeasible. Second, the definition of a defect in AM can be ever-changing depending on the process parameters, environmental conditions, time-dependent covariant, and other hard-to-measured or even unmeasurable variables. To solve this issue, we propose a weakly supervised method we call Isometric Approximation (IsoAp) to address the issues of varying defects and large amounts of needed data. This method requires less data collection and labeling processes, along with ease of re-training for the neural network to adapt to different defect classification tasks.
Project partner: Boeing Company, Lincoln Electric