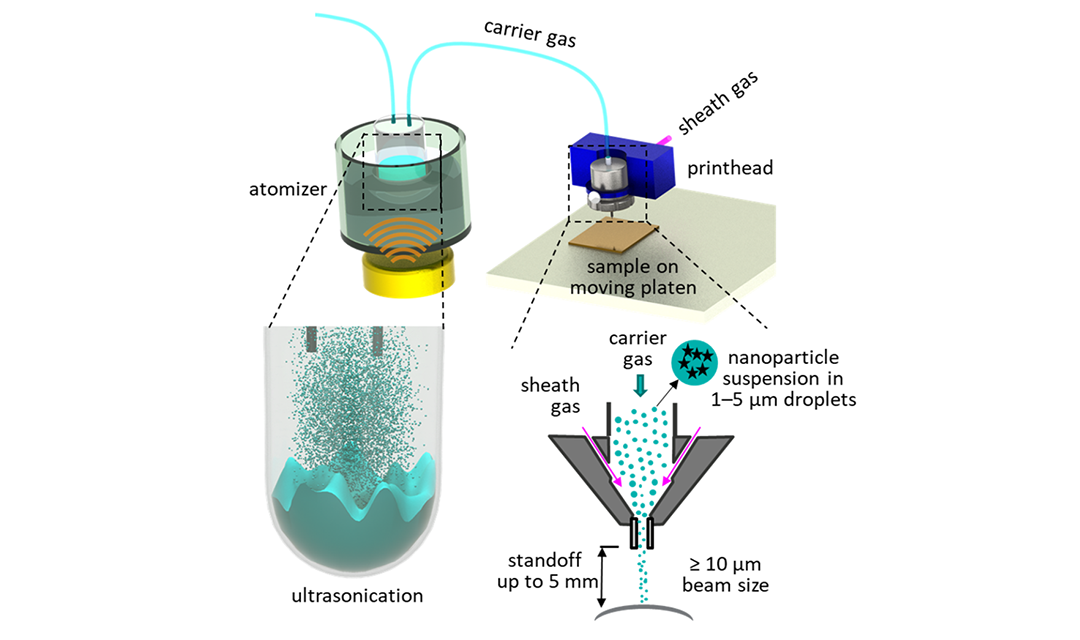
This project aims to develop a probabilistic digital twin (DT) framework that is applied to process control of aerosol jet printing (AJP) of metals, polymers and functional materials to complete high-quality metal interconnects.
Intended project outcomes are to provide tighter tolerances on printed linewidths, leading to tighter tolerances on performance of printed interconnect and sensors, and to accelerate the introduction of new materials for use in AJP through automated recommendations of process recipes based on accumulated modeling across existing materials sets.
The first steps to accomplishing these goals are to characterize and model the process window over extended run times, from run to run, and over different material sets. A unified schema that defines and supports interaction between the AJP model, simulation, state estimator, and data collection will be established, and the state estimator is being developed to dynamically adjust the model as informed by the run-time machine data.
A machine learning model links run-time optical measurement data to the width and height of the 3D printed traces. As a Bayesian dynamic network, the estimator will update the AJP state based on prior history, current model parameters, control commands, measured data and extracted features, and simulation prediction. Experimental results will demonstrate AJP quality and repeatability improvements.