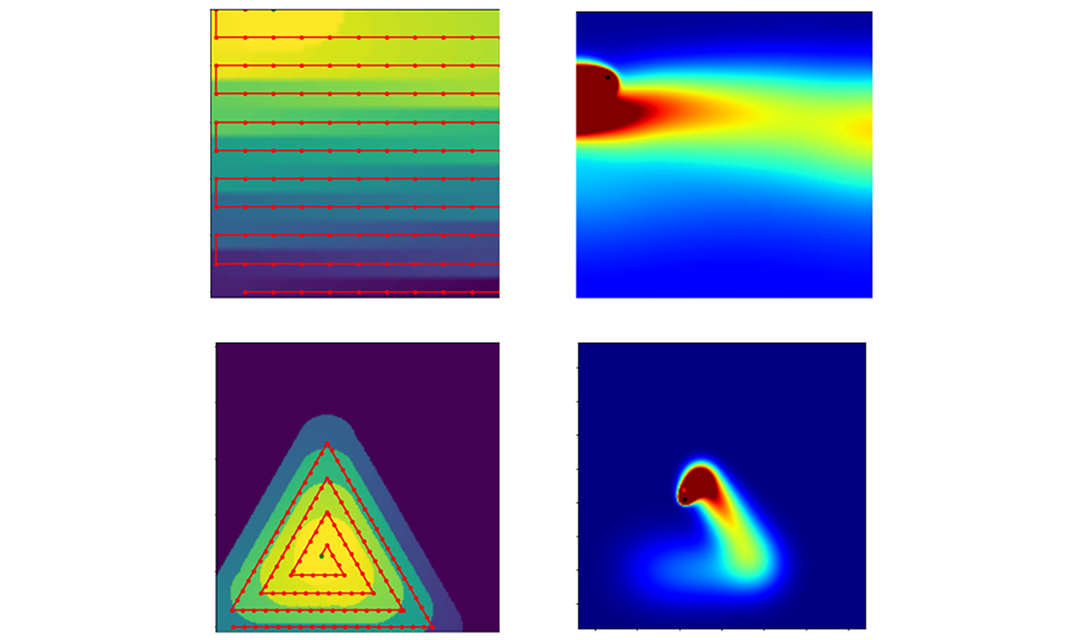
This project applies recent advances in the field of Deep Reinforcement Learning (DRL) to develop sophisticated control strategies for laser powder bed fusion. LPBF techniques are susceptible to defects caused by the properties of the temperature distribution and the melt pool created during the melting process. Therefore, process parameters must be carefully chosen to reduce the occurrence of these abnormalities in the manufacturing process, and ideally be able to adjust to the changing temperature distribution during the scan trajectory. Classical optimization methods have been used in the past to produce control strategies to reduce the occurrence of mechanical defects, but these methods require severe model order reductions, and are restricted in the amount of data that they are able to process due to computational expense. Evolutionary algorithms, such as deep reinforcement learning, show promise in adapting to previously unseen process parameters and dynamically changing conditions. This project will develop and apply DRL methods to discover sophisticated control policies to stabilize and optimize the thermal properties of the powder bed during the melting process. Specifically, a DRL algorithm will be used to optimize the power provided by the heat source in conjunction with the velocity of the heat source, with the goal of ensuring a consistent melt pool depth, without overheating the substrate. These results will be validated through comparisons with results obtained by classical optimization methods and closed-loop control. Success in this project will provide a method for improving the mechanical properties of objects produced using additive manufacturing.