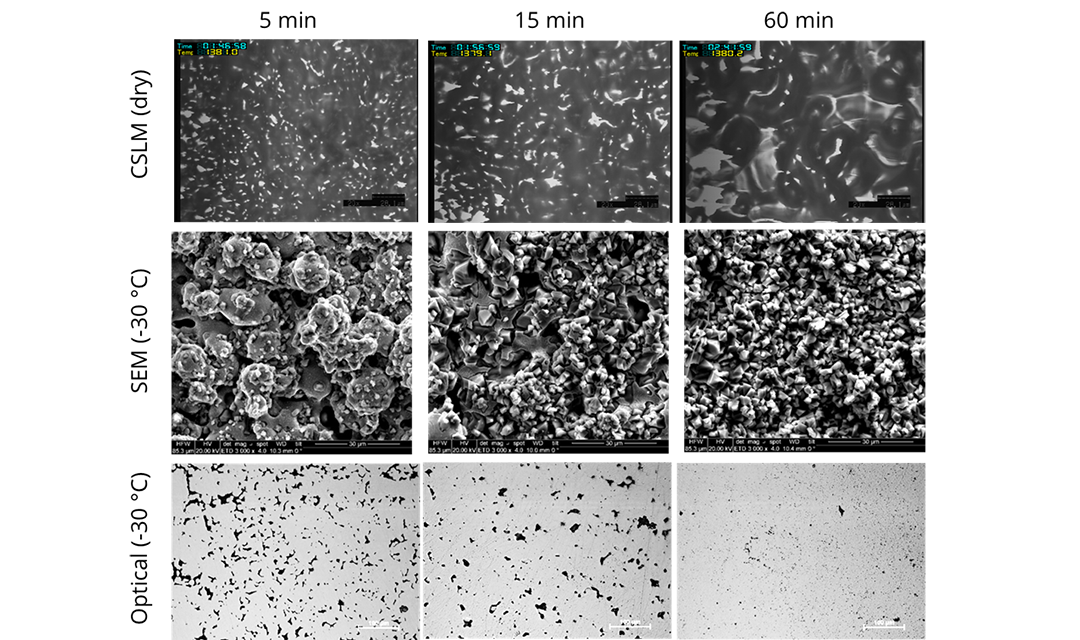
The project aim is to use AI methods to classify images of partially and fully sintered 316L (stainless steel) coupons produced by binder jetting. Production of high-quality metal parts by binder jetting relies on control and quantification of defects. This project will apply an approach to monitoring binder-jet part quality: test coupons ("witness coupons") will be printed and sintered in parallel with parts. The premise is that the test coupons will have quantitatively the same defects (pores and oxides) as the parts. Defect quantification will rely on AI-based methods to process the large volume of defect information that is produced by automated analysis of samples (using scanning electron microscopy with micro-analysis). The objective is a recommended procedure for printing and analyzing witness coupons for rapid quality control on binder-jetted parts. This will support the army modernization goal of a rapid, iterative process of producing new parts. In the work completed for this project, the input images were obtained by high-temperature confocal scanning laser microscopy (CSLM); this method images the surface of the coupon as it is being sintered. Based on visual inspection, the images do change during sintering, so hold promise as a way to directly monitor sintering while it is occurring (on a laboratory scale).
Project partner: The ExOne Company