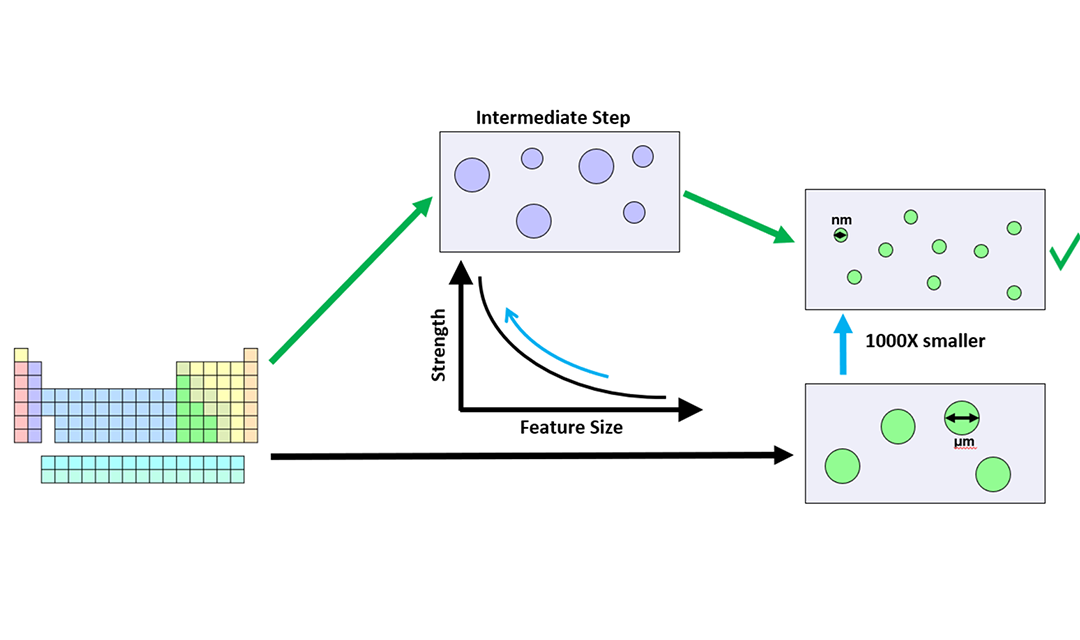
The compositional alloy design for powder bed fusion (PBF) is a complex multi-dimensional multi-constraint optimization problem far beyond the human ability to solve, especially now that printability is as primary a design goal as mechanical performance.
Creating a digital twin by developing efficient integrated computational materials engineering (ICME) techniques has reached considerable maturity for material design in conventional processing. However, we still do not have reliable models for all target properties in PBF manufacturing. More importantly, we remain unable to explore the whole multi-dimensional compositional space.
In this project, advanced ICME, machine learning (ML), and inverse design techniques will be combined to explore the entire design space and discover a global extremum for target combinations of properties. An accelerated experimental workflow will be developed to first, generate required data for unknown parameters and develop unspecified models; second, validate the numerical/analytical predictions; and third, decrease the uncertainty of numerical predictions. A workflow by laser-scanning arc-melted samples will be adapted. Analysis of the uncertainty of this approach for compositional design of 3D-printed samples with various PBF machines and processing parameters of a single machine will be completed. Lastly, the hybrid numerical and experimental workflow will be tested on the newly developed printable, high-temperature, high-strength Al alloys to scale up the design of this novel alloy for various combinations of properties in different industrial applications.