Distributed artificial intelligence at the edge and beyond
Jacob Williamson-Rea
Mar 3, 2021

However, AI is currently limited by the need for massive data centers and centralized architectures, as well as the need to move this data to algorithms. To overcome this key limitation, over the next 5-10 years, AI will evolve from today’s highly structured, controlled, and centralized architecture to a more flexible, adaptive, and distributed network of devices. This transformation, called “AI Fusion,” will bring algorithms to data, instead of vice versa, made possible by algorithmic agility and autonomous data discovery.
Algorithms will ultimately travel to the data, whether this data is collected at the edge or on-platform. This drastically reduces the need for high-bandwidth connectivity, which is required to transport massive data sets, and eliminates any potential sacrifice of the data’s security and privacy.
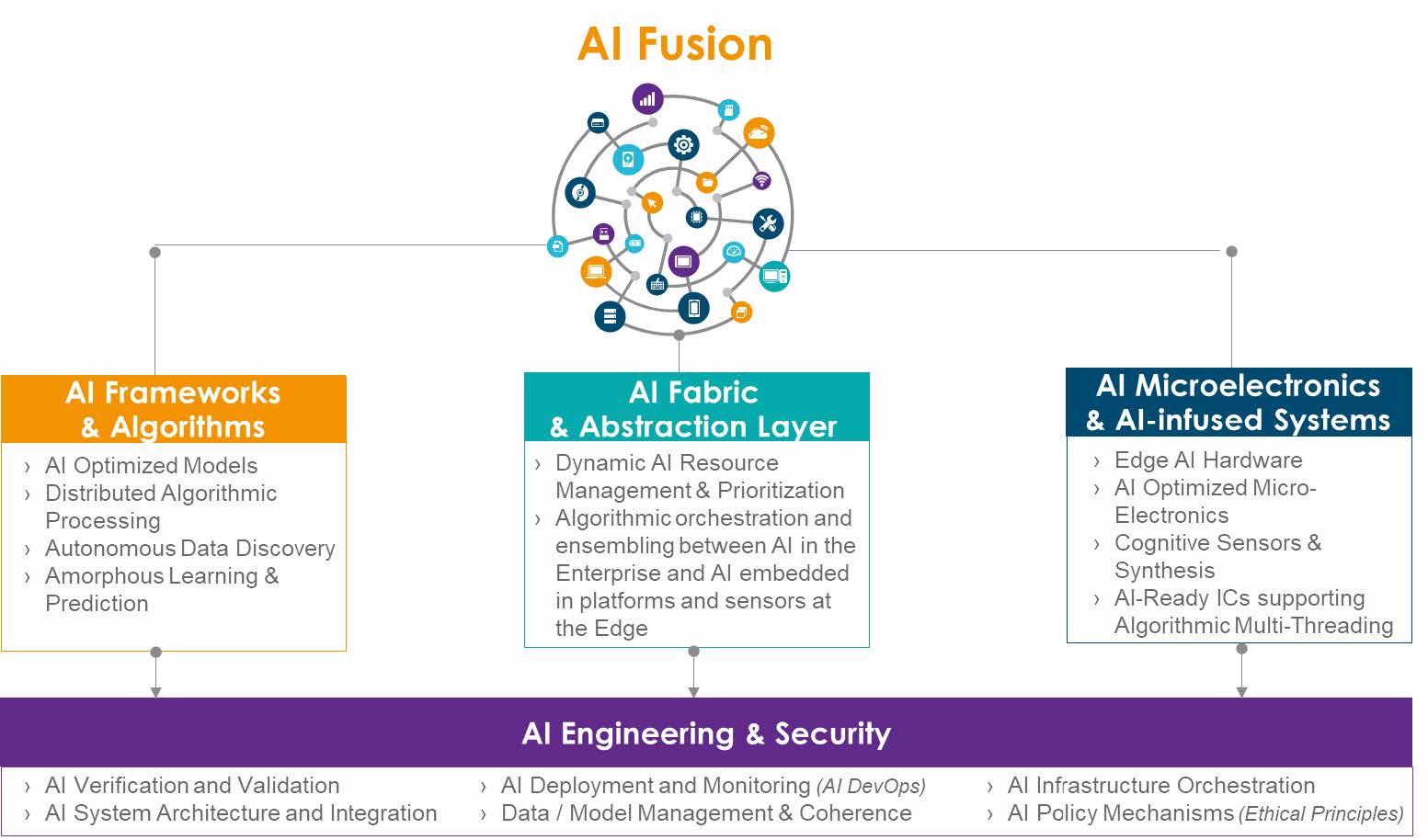
Precursors to AI Fusion are emerging in federated learning and microelectronics optimized for neural networks, but the full potential AI Fusion will require advances in four key research areas: AI frameworks and algorithms, AI fabric and abstraction, AI microelectronics and AI-infused systems, and AI engineering and security.
- AI Frameworks & Algorithms—Algorithmic agility and distributed algorithmic processing will require new AI algorithms that extend autonomous discovery and processing of disparate data well beyond the current limits of federated learning, information theory, and meta learning. With these advances, the cloud will serve as an enabler for algorithmic mapping and orchestration between the enterprise and many, disparate systems and devices at the edge.
- AI Fabric & Abstraction Layer— An AI Fabric is critical to facilitate distributed algorithmic processing between the enterprise and the edge. Extensive research into novel mathematical theorems and frameworks, based on stochastic analysis and models of distributed systems, is necessary to ensure the performance, prioritization, scheduling, resource allocation, and security of the new AI algorithms—especially with the very dynamic and opportunistic communications associated with military operations in contested environments.
- AI Microelectronics & AI-Infused Systems—Supporting dynamic and autonomous AI processing at the edge and on-platform will require extensive research into microelectronics architectures, processing, and connectivity well beyond today’s focus on 3D architectures and Systems on a Chip (SoC). More importantly, extensive research in the co-design of the microelectronics with the AI Algorithms & Frameworks and the AI Fabric is needed to enable scalable training, inferencing, and prediction at the edge and to support algorithmic multi-threading on a single embedded chip or AI-infused system or sensor on-platform.
- AI Engineering & Security—With the exponential increase in AI applications and deployments, extensive research is needed to establish a new ‘AI Engineering’ discipline for developing resilient, reliable, and se-cure AI systems. Simply put, AI Engineering and Security brings ‘confidence in capability’—knowing when AI systems are going to work and when to fix them—across the AI Fusion research thrusts, a task made more difficult as we embrace algorithmic agility and distributed processing and fuse AI capabilities be-tween the enterprise, the edge, and on-platform AI-infused systems operating across multiple domains.
AI Fusion will transform the AI Stack
In 2016, Carnegie Mellon created the AI Stack blueprint to develop and deploy AI. The premise of the AI Stack is simple—AI isn't just one thing. It's built from technology blocks that work together to enable AI. The AI Stack is a toolbox, and each block houses a set of technologies that scientists and researchers use as they work on new projects and initiatives. Each technology block depends on the support of the blocks beneath, while also enhancing capability in the blocks above.
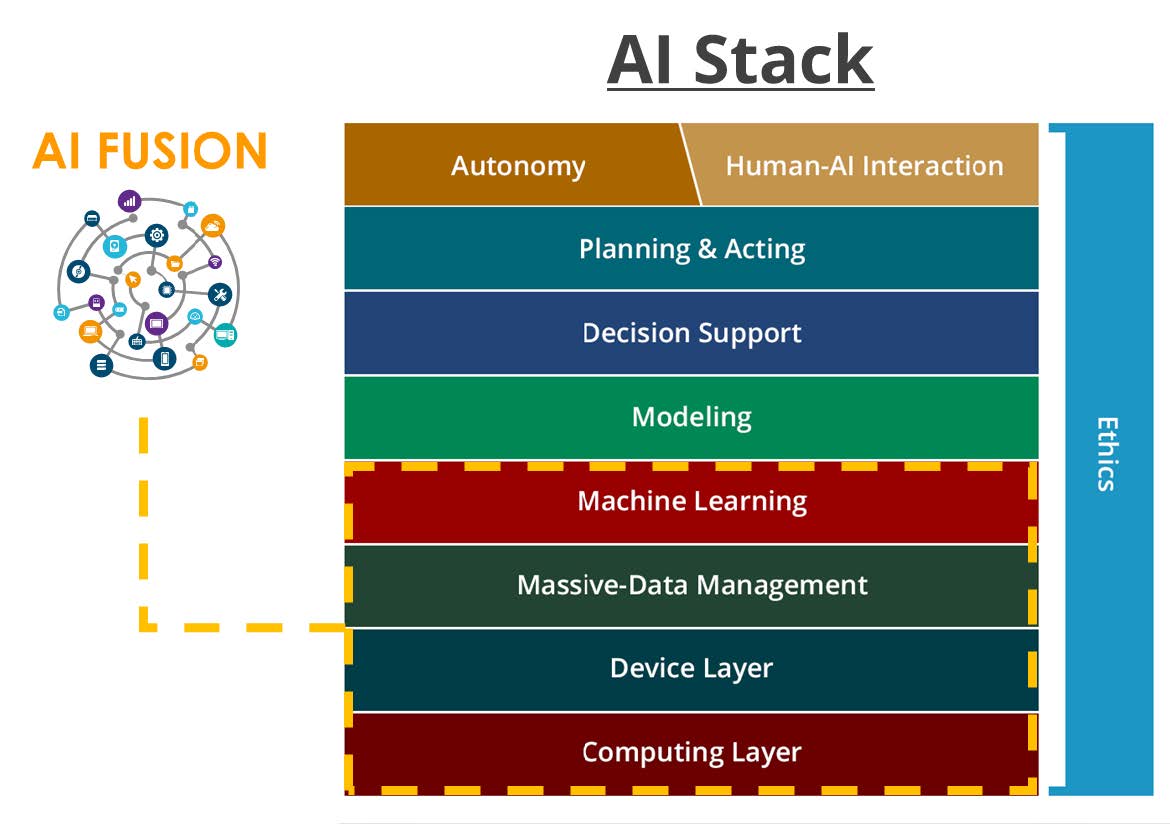
In a traditional, centralized AI architecture, all of the technology blocks would be combined in the cloud or a single enclave to enable AI. When it comes to Distributed AI, AI Fusion will transform the very foundation of the AI Stack by enabling transformational advances, in the above mentioned four research thrusts, which will fuse these capabilities together. This will enable dynamic and autonomous AI processing on the edge or embedded on-platform.
Algorithmic agility and distributed processing will enable AI to perceive and learn in real-time by mirroring these critical AI functions across multiple disparate systems, platforms, sensors, and devices operating at the edge.
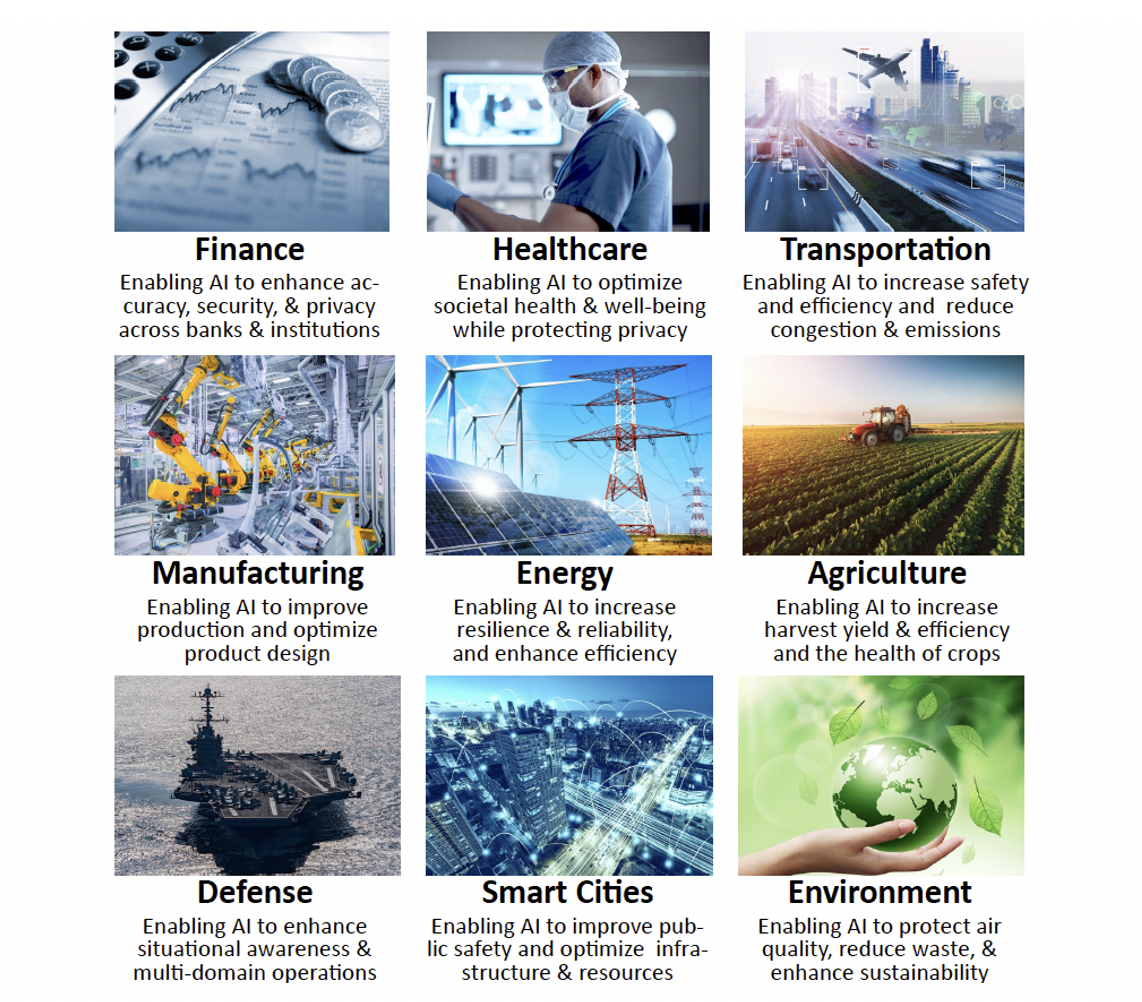
AI applications have accelerated thanks to ongoing advances in computing and data storage, as well as processing capability in the enterprise and the cloud. These same advances are fueling the transformation toward Distributed AI. AI Fusion will enable machine learning to become more scalable and amorphous between the Enterprise and the edge, and to autonomously discover and move to both known and unknown data sources and process in parallel across multiple, disparate platforms, systems, and sensors in real-time. This will enable unparalleled integration of AI across every sector and aspect of out lives to improve productivity, efficiency, safety, and the quality of lifem with applications in:
Faculty interested in contributing to AI Fusion and AI Fusion applications are encouraged to contact ADR Burcu Akinci bakinci@cmu.edu or Matthew Sanfillipo mattsanf@cmu.edu.