Thrust one: Characterizing and predicting rare events in materials systems
A fundamental tenet of materials science is that processing generates the microstructure that mediates material properties. This thrust is built around discovering processing-structure-processing (PSP) relationships for fatigue failure in polycrystalline aerospace alloys, primarily Ti-based systems. In this thrust, we capitalize on computer vision (CV) methods applied to characterize and analyze the multimodal information contained in microstructural images coupled with machine learning (ML) to discover PSP connections.
CMU: Rollett (MSE), Poczos (ML)
AFRL: Shah, Simmons, Payton, Criner, Musinski, Shade, John
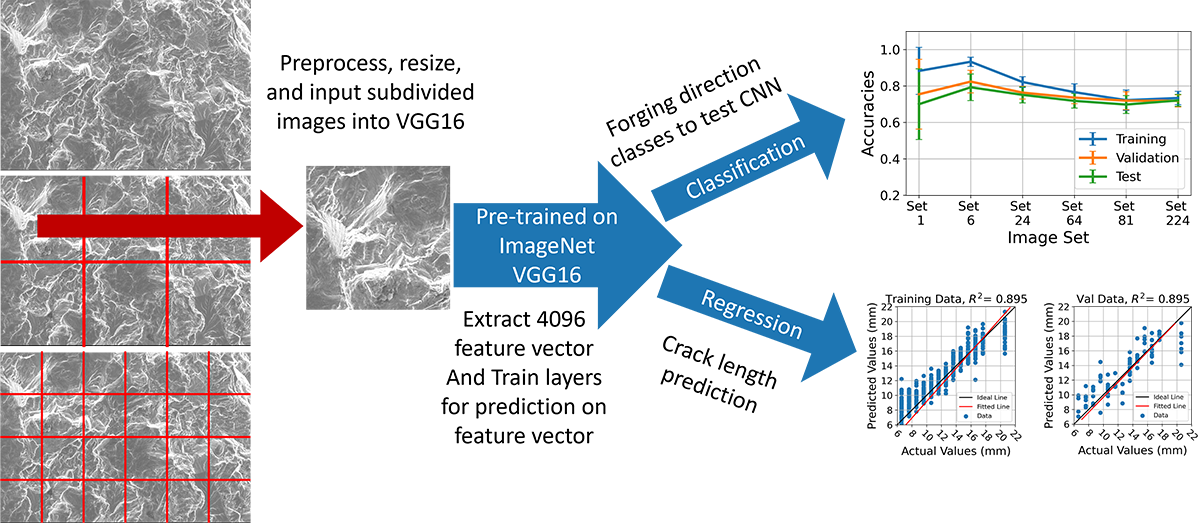
Thrust two: Multimodal materials data fusion
During the past two decades, the field of 3D materials characterization has matured to the point where high quality, large scale, multi-Tbyte data sets can be acquired. To a large extent, these experimental techniques involve scanning every point in a volume without considering whether or not that point will actually provide additional information about the microstructure. In our second research thrust, machine learning and signal processing is being applied to reveal and take advantage of structures in the measured data to dramatically speed up the acquisition process in scanning electron microscopy (SEM), high angle annular dark field (HAADF) tomography in the transmission electron microscope (TEM), and high energy diffraction microscopy (HEDM) at a synchrotron beam line.
CMU: De Graef (MSE), Rohrer (MSE), Rollett (MSE), Chi (ECE)
AFRL: Uchic, Donegan, Shah
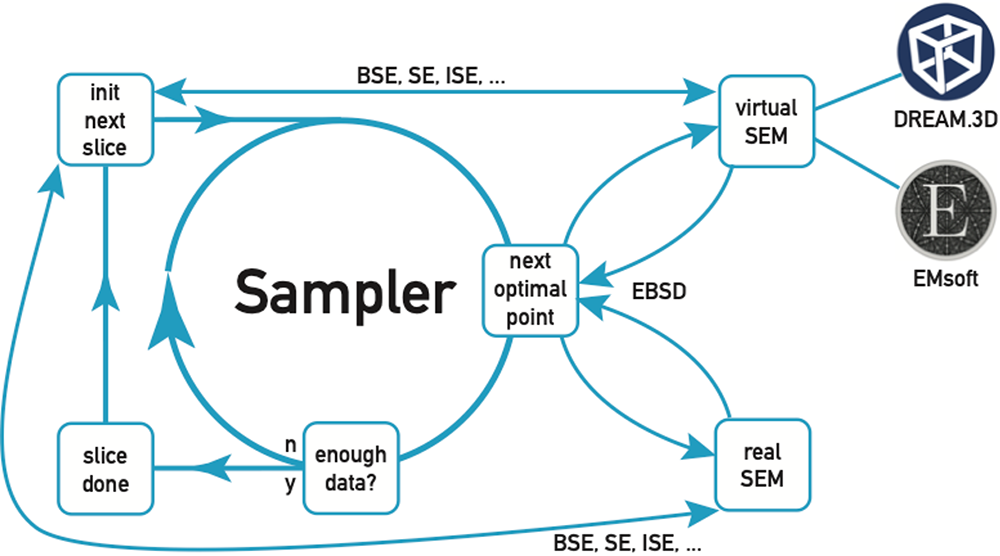
Thrust three: Adaptive experimental design
Design of experiments for materials discovery is currently primarily done by humans in an ad-hoc manner and is prone to human errors, biases, and limitations in navigating complex high-dimensional spaces. Further, since collecting and/or labeling data is often costly, experimental datasets are often small with incomplete data representations. In our third thrust, we develop AI methods that can integrate feedback from prior data, simulations, and expert knowledge to guide subsequent experiments to efficiently search for materials with desired properties, with a focus on oxide materials. The approach integrates with the ARES software package developed at AFRL.
CMU: Jayan (ME), Rohrer (MSE), Singh (ML)
AFRL: Maruyama, Hooper
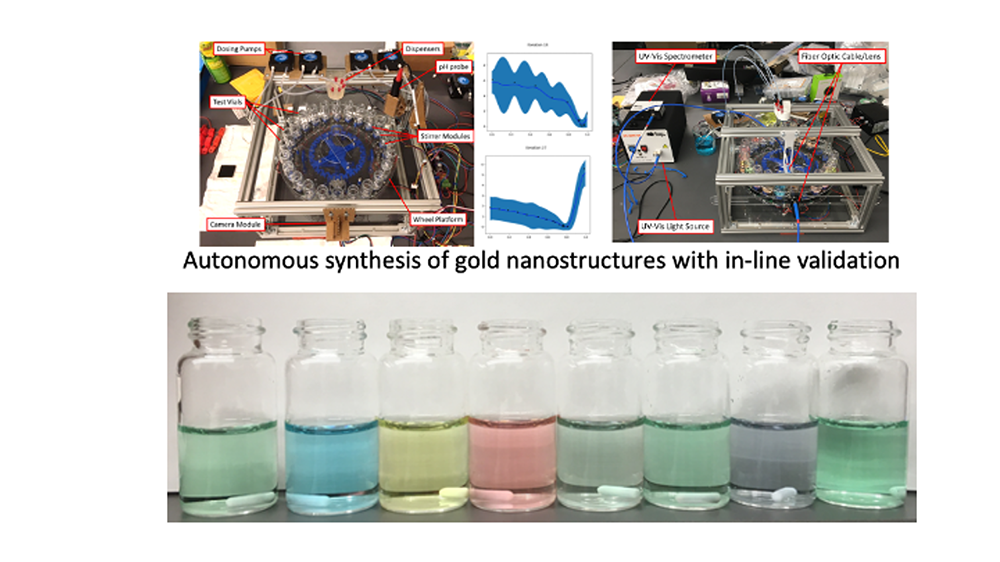
Thrust four: Adaptive materials design in complex environments
Active materials that are capable of organizing "on-command" into superstructures with desired properties promise breakthrough advances across a range of defense and energy related material technologies. A pervasive challenge in the realization of such materials is the vast and interconnected parameter space to be explored. The goal of this thrust is to establish toolsets and protocols for the efficient navigation of large parameter spaces that can be applied to the optimization of complex material systems and their adaptation to evolving constraints. The material testbed is constituted of self-assembled brush particle-based materials that are to be optimized for multiple property combinations.
CMU: Bockstaller (MSE), Kara (ME), Yao (HCII)
AFRL: Harper, Drummy, Buskohl, Gellman, Baldwin
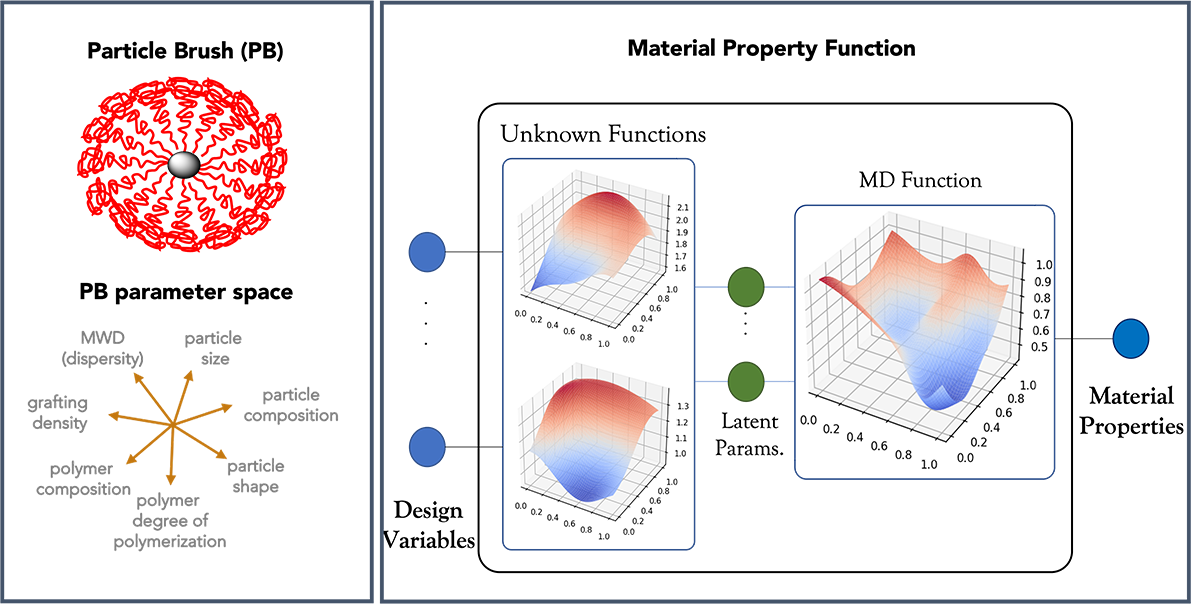
SEED One
AFRL: Luke Baldwin
SEED Two
CMU: Kaushik Dayal (CEE), Carmel Majidi (ME), Michael Vinciguerra (ME)
AFRL: Matthew Grasinger, Dhriti Nepal, Vikas Carshney