Detecting and Stopping Brain Tsunamis with EEG Technology
Jacob Williamson-Rea
Oct 21, 2020
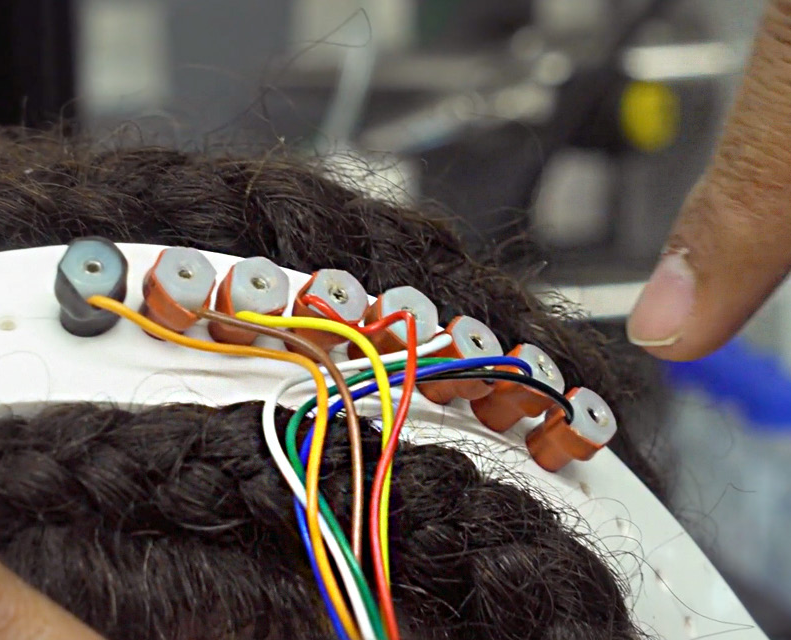
Source: Carnegie Mellon University
Technically referred to as a cortical spreading depolarization (CSD), a brain tsunami moves like a powerful and slow wave across the brain surface. This complex phenomenon has emerged as one of the most reliable indicators of severity of an initial injury, but the tsunami itself can also cause further damage. As part of a vicious feedback loop, the intense spikes of hyperactivity and inactivity can further damage cells, worsening the brain’s condition beyond the initial trauma.
A research team led by Carnegie Mellon University, in collaboration with clinicians at the University of Pittsburgh, is exploring how to detect and minimize additional brain damage. They paired up with Precision Neuroscopics, a Pittsburgh-based startup company, as part of a 2018 PITA project.
“I think detection of worsening brain injuries is a hugely important problem, with no existing solution that actually works,” says Pulkit Grover, associate professor of electrical and computer engineering at Carnegie Mellon University. “Detection of brain tsunamis, and their subsequent suppression, could be a game-changer.”
“We had been exploring electroencephalogram technologies in general, mostly focusing on sensing brain signals noninvasively,” says Shawn Kelly, co-founder of Precision Neuroscopics.
An electroencephalogram (EEG) measures the brain’s electrical activity and ideally could be used to evaluate the extent of an injury. The Carnegie Mellon and Precision Neuroscopics team wanted to do more than just measure the brain’s electrical activity — they wanted to detect brain tsunamis.
Grover, Kelly, and Ashwati Krishnan, a postdoctoral researcher at Carnegie Mellon’s Center for the Neural Basis of Cognition, set out to detect brain tsunamis, along with other researchers in Grover’s lab. “We are designing the algorithms for detecting brain tsunamis,” Grover says. “The techniques I’m exploring include machine learning and signal processing, and I’m also working closely with clinicians to understand their end goals.” This work is led by Ph.D. student Alireza Chamanzar in Grover’s lab.
Meanwhile, Krishnan has been refining the conductive sponge technology, which allows the researchers to administer EEG recordings noninvasively — and at a lower cost than traditional EEG technologies.
“Typically, when researchers use sensors to measure brain activity, they use an electrode gel, which contains ions,” says Krishnan. “The gel allows the brain and the sensors to ‘talk’ to each other.”
However, electrode gels are costly, require extensive setup, and ultimately do not provide a high enough spatial resolution of EEG for detecting additional post-injury damage.
“Ashwati [Krishnan] brought in her drive for low-cost, high-quality biomedical diagnosis and treatment systems,” Grover says. “The entire idea of conductive sponges is her idea, from conceptualization, to design and implementation. She mentored an extremely capable CMU undergraduate, Kalee Rozylowicz, who is now planning to pursue graduate school. Both are co-authors on the primary publications resulting from this work.”
Krishnan says that the partnership with Precision Neuroscopics is crucial in the scaling of their technology, and that partnerships like these are important across the entire field in general.
“I believe that engineering is a field where you can’t just prove that something works once in a research lab,” she says. “Engineers need to think about applying technology, because engineering shines when people actually use it. I appreciate this relationship with Precision Neuroscopics because there is an immediate possibility for taking what we do in the lab and then directly translating that to a larger scale.”
“Precision Neuroscopics have been amazing collaborators,” Grover says. “They helped us set up an entirely new lab, provided us feedback and expertise at various stages, and it was great to see Pittsburgh graduates find jobs at Precision Neuroscopics, including some from our lab.”
“The successful PITA partnership led to increased funding,” Grover says. Including a DARPA award of about $2.4 million, as well as a grant from the Center for Machine Learning and Health at Carnegie Mellon University, which will be used to further develop and refine the technology.